Visualizing the Invisible with MEG (video and transcript)
In this interview, the directors of the new Qualcomm Institute (QI) Magnetoencephalography (MEG) Center Roland Lee, M.D, and Mingxiong Huang, Ph.D., speak on topics from the underpinnings of the advanced brain imaging technique to the making of the new facility and MEG’s contributions to research and patient care. Leading the conversation is Ramesh Rao, Ph.D., QI director and electrical and computer engineering (ECE) professor in the Jacobs School of Engineering at UC San Diego.
The transcript below (edited for clarity) contains the following sections:
- Introduction
- Journey to UC San Diego
- Electric and Magnetic Fields in the Brain
- Faint Signals in a Noisy Environment
- A Tool for Diagnosis and Treatment
- Looking into the Future
- State-of-the-Art Machine, State-of-the-Art Chamber
- The Power of Partnerships
INTRODUCTION
RAMESH RAO: Hi, I’m Ramesh Rao, director of the Qualcomm Institute, and I wanted to share with you today an exciting new development for us here at the Qualcomm Institute. It’s a new facility which houses a unique new instrument called the MEG machine. And I’m going to invite my colleagues, Dr. Roland Lee and Dr. Mingxiong Huang, to describe what an MEG machine is, what it does, and what is its significance.
ROLAND LEE: I’m the director, with Mingxiong, of the MEG facility now newly located to Qualcomm Institute on the UCSD campus. I’m a physician and professor of radiology and neuroradiology at UCSD and the V.A. Medical Center. MEG stands for “magnetoencephalography.” “Magneto,” magnetic fields; “encephalography,” the measurement of the brain. So this instrument measures the magnetic fields emitted by the subject’s brain. The image unit will be largely used for research here at Qualcomm Institute. There are many researchers from all different fields, and now we’re part of that facility. So we’ll be collaborating with researchers from across the campus coming together here, at QI, both in the medical field and in the sciences. It also will be used to treat, to diagnose, patients as well with diseases such as epilepsy.
MINGXIONG HUANG: I’m a professor in radiology and also research scientist in the VA in San Diego. So my training is biomedical physics, and I’m co-director of the MEG with Roland. My major interest is to develop MEG source imaging algorithms, so we can generate high-resolution MEG source images and also apply those algorithms to different brain applications for both clinical and research projects. MEG is a functional imaging technology and it’s noninvasive. The combination of sensitive sensor and the magnetically shielded room allows us to measure the tiny magnetic field when neurons are talking to each other. So we encourage all PIs who have interest in brain function to work with us.
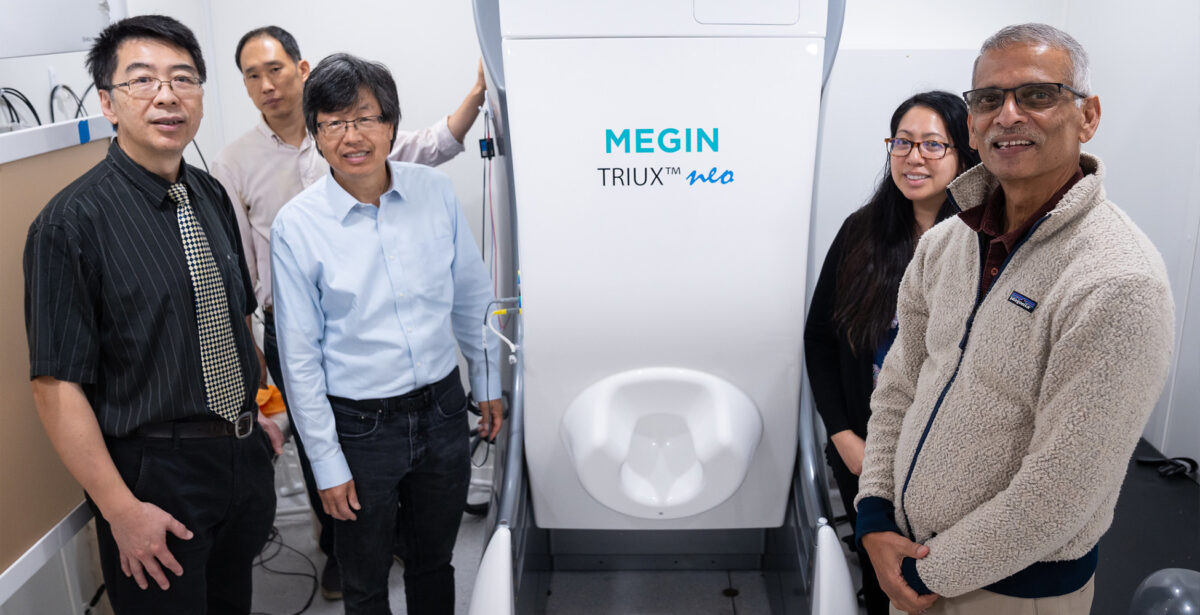
RAO: Thanks for introducing yourself. Now let’s do a deeper dive.
JOURNEY TO UC SAN DIEGO
RAO: Roland, I thought I could start by asking you to describe your journey. What brought you to where you are today? What attracted you to this field, and what are the key moments that defined the trajectory that you took?
LEE: Okay, thank you. I started out as a student of physics. I got my bachelor’s in physics at Caltech, and then I went on to do graduate school in low temperature physics at Berkeley. Then I realized that, in my particular life and situation, it would be good to go into the medical field.
And so, as part of the training in medicine, after med school you do residency. And then I found that there are ways you can use physics to help look at the body and in particular the brain. So I became a neuroradiologist and worked at Johns Hopkins as the head of neuro-MRI. But then I realized there was an opportunity to study this instrument called magnetoencephalography at the University of New Mexico. There were very few at that time. That was one of the premiere ones. So I was recruited to New Mexico to be the director of the magnetoencephalography unit, which had just been recently established there. So I was doing clinical work in neuroradiology, but also spending most of my time doing clinical and research on the MEG unit there.
That’s where I met Mingxiong, who is the main scientist and one of the top MEG scientists in the world on this. We just happened to form a natural team. So we were there for a few years together, and then, in 2004, we came to UCSD.
RAO: Wonderful. So MEG machines were around when you were starting out as an undergraduate student in physics?
LEE: When I was in physics, there weren’t any MEG units yet. They were just getting started in the maybe 1990s to do the whole-head MEG. And so it was really fortuitous at the time. I was getting interested, just as they were just making the first whole-head MEG units. We had one of the earliest ones in New Mexico.
RAO: Amazing. Mingxiong, what got you going down this path, and what was your educational journey in research?
HUANG: I came to the US actually in the early ’90s, after the Tiananmen Square Massacre in 1989. Actually, I lost two close friends in the Tiananmen Square Massacre. So my wife and I came to the US in the early ’90s, to study physics for my PhD topic.
In my last couple of years there, I got to know a faculty member. His name was Ron Aaron, and he introduced me to this field of MEG. He did a sabbatical in the biophysics group in Los Alamos National Lab. Shortly after I got my Ph.D., and my topic was focused on the forward and inverse algorithm and modeling for MEG, I had a postdoc position at Los Alamos. I spent a couple of years there working with some top physicists and also neuroscientists on MEG, including Dr. Ed Flynn. Dr. Edward Flynn is one of the founding fathers for MEG, along with David Cohen.

So during that time I got to know Dr. Roland Lee. After I finished my Ph.D. training, I became a junior faculty member at the University of New Mexico and also the VA hospital there. During that time, I continued to develop the MEG forward and inverse modeling techniques, and had the idea that we’re going to make MEG source imaging with high resolution. MEG is a functional imaging technology with a millisecond temporal resolution. Also, on the cortical level, with a good algorithm you can actually have a spatial resolution of two or three millimeters. So it’s an excellent combination of high resolution in time and space. So during that time I continued working on the algorithm development and also applying those technologies to different applications, including traumatic brain injury and post-traumatic stress disorder. Roland and I moved from UNM to UCSD in 2004. We established a new lab here and that actually allowed me to have a lot of collaborations with some excellent collaborators here and also in the VA San Diego.
RAO: It’s interesting you both arrived here in 2004, going back to the founding of this institute. You know, these Institutes for Science Innovation were created at the very end of 2000. And the physical facility, Atkinson Hall that we are in, was completed in about 2006. So you roughly arrived a couple of years before this facility was built.
ELECTRIC AND MAGNETIC FIELDS IN THE BRAIN
RAO: The brain is a fascinating organ, regardless of who you are or what your background is. The fact that we can think about our own brain is a unique aspect of what makes us human. But from an electrical engineering perspective, from an electromagnetics perspective, I wonder if you could share with us how you think of the brain as an electromagnetic source. What’s happening inside the brain from an electromagnetics point of view? Roland.
LEE: As a radiologist, we take pictures of the structures we’re looking at. So we take a picture of the knee, shoulder joint or your heart, which is a moving organ, or the brain. Now, for some organs, just looking at the picture of it is pretty good. For example, the heart is a pump, so you can tell if it’s working well or not because you can see it pumping. But the brain does not move, and it’s hard to tell how the brain’s working by looking at a picture of it. Now, as radiologists, we’re very good at seeing diseases like a mass lesion or destruction of the brain, where the brain starts getting bloody and so forth. But that’s not really the function of the brain. In order to do that, you really need to look at the electromagnetic properties of the brain, such as on EEG or, even better, MEG, because the electric fields are distorted by the skull and the magnetic fields aren’t.
RAO: So tell us a little bit more. Why is it that electric fields are distorted but not magnetic fields as much?
LEE: It’s just a property of matter. We see electric fields all the time. If you’re going across a carpet, you can create electric sparks. You can get charged on a winter’s day, and you can have your hair stand up. But what you realize is that other things can disturb those electric fields. So, for example, I have a comb and I have my electric fields, and my hair’s standing up. And if I put something between there, I can block the electric fields and change it. Whereas, with magnetic fields, that’s not the case.
RAO: So the magnetic signals that you’re trying to pick up with MEG are extremely faint. And there’s a lot that goes into dealing with that, from building the chamber to doing the analysis. But I was wondering if the magnetic field is just a byproduct of the electrical activity in the brain, or does the magnetic field play an essential role in some way? Does the brain take advantage of this magnetic field? Does it help different regions of the brain connect with each other? Is this just a consequence of electromagnetism that you have a magnetic field and an electric field?
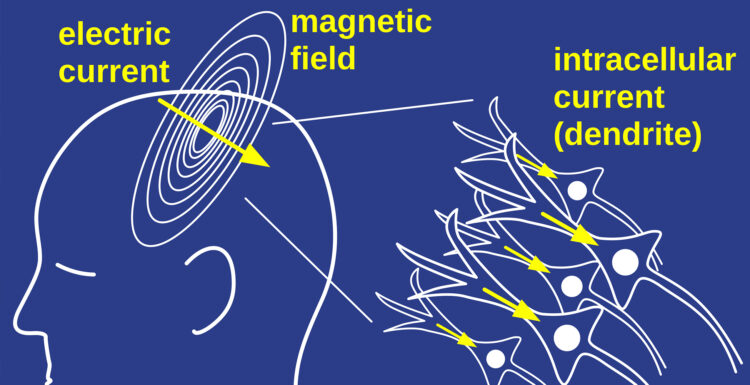
LEE: My feeling: it’s just a consequence of the electric fields. The electric currents run down the axons with neurons. The neurons are nerve cells. And then they have projections, like wires, that attach them, billions of them. Running in concert, though, is this electric field, these currents going from neuron to neuron. The currents are the main things that are driving the activity. But every current has both electric and magnetic fields. So it’s an indispensable and unavoidable consequence of the currents that we have these electric fields that we measure by EEG, or the magnetic fields that we measure by MEG.
RAO: That’s interesting. Now, there are other modalities that you sometimes use in conjunction with imaging, like MRI and others?
LEE: Absolutely. We have to have an MRI of the brain to show that person’s brain and then see where the magnetic activity is located, not just in space, but within the brain. So you always have an MRI first, and then we can fuse the 3D location of the pictures of the brain from the MRI with the 3D locations of the source activity, which Mingxiong’s programs will decipher.
FAINT SIGNALS IN A NOISY ENVIRONMENT
RAO: So on that note, Mingxiong, you’re picking up these really faint signals in a noisy environment and you’re trying to identify the locations, the sources, of these signals. So there’s a great deal of signal processing, a great deal of modeling work, that probably has to be done for you to be effective. Could you elaborate on that?
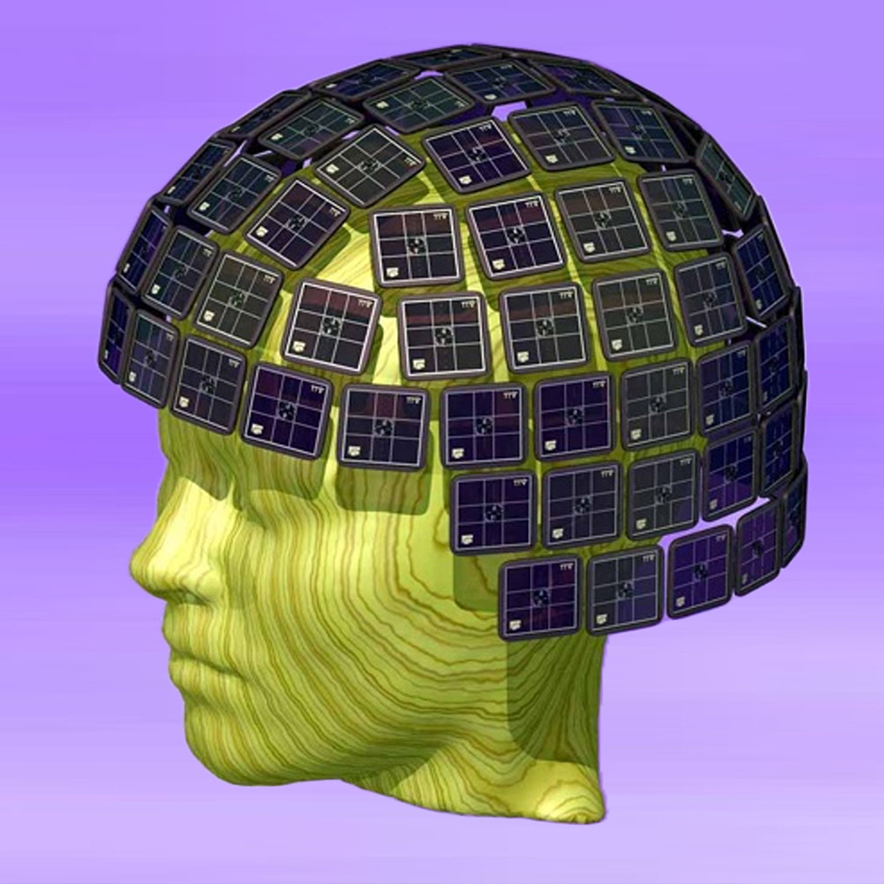
HUANG: From the signal point of view, the signal is, as we mentioned, extremely weak. We talk about the evoked MEG field in the order of a few hundred femtotesla. One femtotesla is 10(-15) tesla. So, number one, to make the signal measurable, we need a very sensitive detector. The one we are using here is a new generation of the MEG system that uses the so-called SQUID (superconducting quantum interference device), that picks up the signal from the brain, which is extremely weak.
Another technology we need to apply is to block the environment’s electric magnetic noise. We need to place the MEG machine, with all the sensors, into a magnetic shielded room. So the one we have over here at QI in particular, uniquely, has a six-layer shielding room—one of the best shielding rooms in the whole world for MEG. So that allows the sensor, the SQUID sensor, inside the room to be able to pick up the brain signals. For the SQUID sensor to be functioning, we need to cool it down to liquid helium temperature. That’s why we need to put the sensors inside liquid helium.
But, back to your second question, to make the source imaging of the MEG, we must solve the MEG forward and inverse problem. The forward problem is: Assuming we know where the source locations are, we know the connectivity profile of the head, the head shape and so on—
RAO: Based on the MRI scan?
HUANG: Based on the MR [magnetic resonance]. The most important layer of the boundary is the inner skull surface. That’s most important because the skull connectivity is extremely low compared to the brain tissue or the scalp. The conductivity of the skull is about one 80th, even a 100th, of the connectivity of the brain signal. So it’s almost like an insulator. The vast majority of the electric current is confined within the skull. So we need to actually trace the surface of the skull to make good MEG forward modeling.
RAO: And how many sensor locations are you doing this modeling for?
HUANG: In our new MEG machine, we have 306 sensors. And 204 of them are so-called gradiometers. They have two coils, like an “A” shape, but with the wires in opposite directions. One loop goes clockwise and the other goes counterclockwise. With the two loops, A minus B; any artifact far away from the brain cancels out. The local fields of the brain are preserved. The other 102 sensors are magnetometers. They’re just one coil, without cancellation. So a combination allows us to have good cancellation of the noise and in the meantime allows pick up of some deep signal. The magnetometer does less cancellation; it can pick up a deep signal.
So when we solve the MEG forward problem, then we can actually solve the inverse problem in a second step. In the inverse problem, assume you take some measurements in a central domain. How do you make images of the sources inside the brain? We are measuring the neuronal current in the dendrite synapses. As Roland mentioned, EEG spatial resolution is quite limited, partly because the skull is like an insulator. Everything is through the skull, and the electric signals are smeared out. In MEG, there’s almost no distortion to go through the skull. So when we solve the MEG inverse problem, we get in the location of the dendrite, the neuron firing. When we solve the inverse problem, there are different hypotheses for the MEG. The one we are using is a spatial-temporal L-1 norm, which gives us a high-resolution sparse solution.
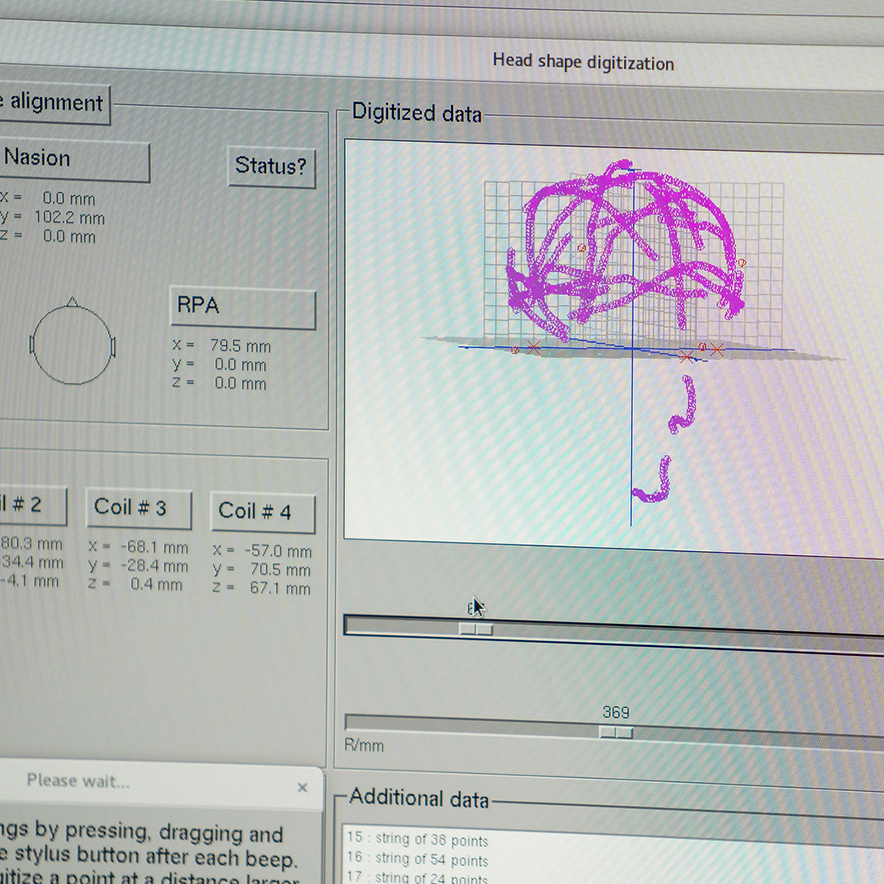
RAO: So a great deal of computation is involved in this. Is this a computationally burdensome exercise to process the 300-plus channels and do the source localization?
HUANG: Yes, there are different types of MEG source imaging or inverse problems. For some of them, actually, computational costs are actually relatively low. But when we talk about high-resolution imaging, like with the L1 minimum norm, the computational costs can be high. Typically when you’re dealing with 306 sensors, that’s the sensor domain. The other domain is the source grid. We have divided the entire brain into a large grid, maybe with 10,000, 50,000, electric current dipoles. So you are dealing with a high-resolution source grid. So the computational cost for a nonlinear optimizer, like VESTEL, can be high. On top of that, a project we’re dealing with is the brain-computer interface. In those projects, we’re dealing with a large neural network, artificial neural network, and to solve those problems we need a lot of computational power.
RAO: So there is this question of the ground truth, right? So you computationally try to do the source localization. How do you ultimately know if you got it right? Maybe that crosses over into medical applications of what you do with this imaging data. Are there methods that help you figure out if the L1 norm minimization that you use actually does produce the desired accuracy? How does one go about achieving that?
HUANG: That’s a great question. First of all, there’s animal research, mainly from Dr. Yoshio Okada’s lab. They used the animal model of pigs to actually verify, in a lot of cases, the brain’s electric activity is quite sparse. We look within each cortical column, with maybe a few hundred thousand neurons. So for MEG and also scalp EEG, we don’t have the sensitivity for single neurons. We look at each cortical column with a few hundred thousand neurons. When they fire synchronously, there’s generally a measurable MEG or EEG signal. If you look at the spatial extent of those sources, they are in the order of millimeters. So there’s animal knowledge and evidence from patients that support this sparse solution that the L1 norm is based upon.
LEE: I can elaborate on what Mingxiong said. So the simplest way to a solution is just single dipole. You can assume that, at one point in time, just one point in space best summarizes the activity.
RAO: This point in space being in the brain?
LEE: That’s right. This is a good example. You take the median nerve. You shock your wrist. That goes to a certain part of the brain that is the ground truth. We know from 100 years of brain surgery and many tests; we know where that spot in the brain is that corresponds to the sensation that you feel when you shock your wrist. That’s well known, and every neuroradiologist can look in at a brain MRI and say, “This is the point where the median nerve is.” There’s a central sulcus, a groove in the brain, centrally. And it’s on the back of that that groove. Similarly, if I move my finger, I can localize what part of brain is happening; 20 milliseconds before my finger goes, I’ll see a part in the brain activates. That’s called motor. That’s exactly on the front of that groove. That’s ground truth. That’s unequivocal.
RAO: So that’s a cross-check on whether the algorithm is doing a good job.
LEE: Exactly. And then when we do the L1 minimum norm, it’s no longer one point. It’s more sophisticated. It gives a patch. It always gives the same spot centered on that point.
A TOOL FOR DIAGNOSIS AND TREATMENT
RAO: So part of what you are doing in radiology is identifying regions that might need attention. But what happens after that, in specific instances like epilepsy and other conditions.
LEE: So for clinical use, for taking care of patients, the most common use of the MEG is in epilepsy. Basically, epilepsy is an electromagnetic disturbance in your brain. So the way the brain works is through currents that cause your thoughts and cause your hands and everything to move. So in epilepsy those currents go haywire. Unfortunately, instead of being nice and orderly, doing things in an orderly fashion, it’s like a thunderstorm in your brain, like one part of your brain or multiple parts remaining just go crazy. That’s an ideal use for MEG, because the signal’s very high. It’s easy to see those activities, and it’s usually basically one spot. At one instant in time, it’s one spot. Now, there may be other spots that propagate and so forth. But if you look at signals of the brain, you’ll see, all of a sudden, these spikes going very high signal-to-noise.
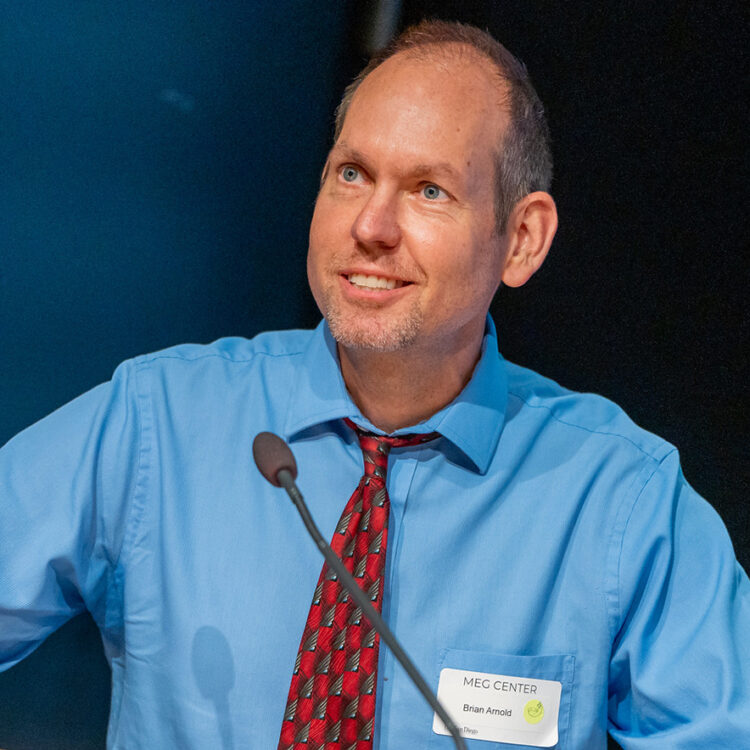
So it’s very easy to localize those, and that tells you where in the brain we have the problem. That’s very important. The good thing about epilepsy is, most of the time, maybe for 70% of patients, you don’t need to know where it’s happening. You know the patient’s having epilepsy because they either pass out or they have these shaking episodes that people know about. But most of the time that can be cured with drugs. Neurology and epileptologists have been very successful at creating drugs that will solve the problem. If you can solve the problem by taking a pill or pills, you don’t need to do a very big workup. Just take the pill. You can function, you can safely drive and go about your life.
What we use the MEG for, and what’s complicated, is the about one-third of patients who are not cured by taking a pill or pills. Epilepsy’s very disruptive to the patient’s life. You cannot safely drive. And it’s not a good condition. It’s a degenerative condition; it gets worse over time if it’s not treated. So, since the pills don’t work, the other treatments are various types of surgery. If you find out there’s one part of the brain that’s acting crazy, if you know where that is, you can surgically remove it. And surgeons have been very good, working with neurologists for years, at taking out certain parts of the brain safely and effectively. There are new treatments now where you can put in a laser beam and just zap it without cutting it out, or else you can put a wire in and measure when the activity is going bad and then zap the brain and that will quiet it down. Anyway, it all hinges on knowing exactly where the abnormal activity is, and MEG is very helpful to do that.
EEG, which was used for a century, can tell you roughly. You know it’s somewhere on the right side of the head, but you can’t see where. With MEG, the skull is transparent. So then I can tell you within just a few millimeters where the activity is. That’s much more helpful to the surgeon. And, when compared with the MRI, a lot of times I can see a lesion. As a radiologist, I can see the lesion in the brain that corresponds to that point. Then it’s great: “I have a bad part of the brain; I can see it looks abnormal; the spikes are coming from there.” That is really what will help the patient’s’ treatment.
RAO: So I understand the connection with epilepsy, now that you’ve explained it. How does it work in TBI and other cases? Is it roughly the same? Are you attempting to go in and modify parts of the brain structure?
LEE: OK, this is a work in progress. Let’s talk about different kinds of TBI, traumatic brain injury. Some types of traumatic brain injury, it’s just very obvious, unfortunately. So if you’re in a bad car accident or something and your brain gets smashed, you’re bleeding in your brain and parts of brain are damaged. We don’t need functional imaging to see that. If you look at an MRI, as we radiologists do every day, we can see blood in the brain; we see a piece of brain that’s been damaged by smacking against the skull. I know where the injury is. Again, we work with the neurosurgeons. A lot of times, there’s nothing you can do to treat it. Or, if it’s a big bleed, then they can take away the blood. The diagnosis is simple when it’s very severe.
Fortunately, most of the time you have brain injury, it’s not that severe. So when you do an MRI or CT scan of the brain, it’s normal—which is good, OK—but something’s wrong. That’s called a concussion. And, in the news, people now know about concussions. It happens to not only professional football players, but also our children, on the football field. It happens when you get in a car accident. Most of the time, fortunately, it’s not bad enough to cause bleeding in the brain or smashing of the brain, but just something’s wrong. But when I do an MRI and CT scan, I can’t see it. Does that mean the patient’s normal? No. It would be good if we could actually have objective evidence that something is wrong.
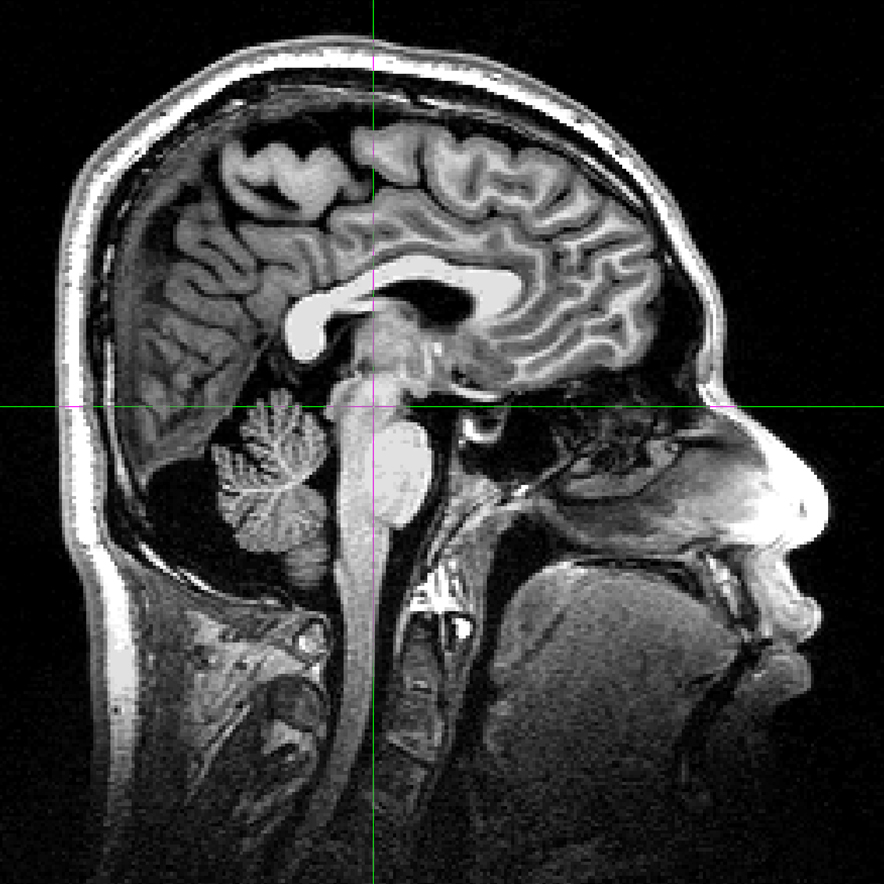
Now, most of time, concussions just get better on their own, over weeks or sometimes longer, sometimes shorter. Fortunately, the brain—and the body—heals itself. But there are cases where it doesn’t get better. Then, first of all, you want to know is this real or not. Is there actually objective evidence that there’s something abnormal?
So that’s another value of MEG. We don’t only look for spikes or other things, we also can see the brain rhythms. So the brain has normal rhythms that, again, we know about because of a century of EEG. We know people have heard of alpha waves. That’s normal. Alpha waves are strong in the back of your head. They get stronger when you close your eyes, and so forth. But that’s normal. There are other frequencies that are high frequency. Alpha waves are like 8 to 13 cycles per second. But we also know from studies of people that you don’t normally have low frequencies. One to four cycles per second in an awake, alert adult, you should not have that. So we are using that—and EEG also uses that—to objectively prove that something is wrong with your brain. So even though the CT scan and MRI are normal, by measuring the MEG, I can find a lot of slow waves in an adult and then say, “That is not normal.” How do we know that’s not normal? Because we’ve done millions of EEGs and many thousands of MEGs, and we know that normal adults just don’t have that.
RAO: So, on that note, having again ground truth on what is normal is helpful. And you have over the years gathered quite a collection of recordings, right?
LEE: That’s right.
RAO: Do you want to share a little bit more about that?
LEE: That’s right. Mingxiong can go into more in detail. With research grants, we have funding to scan many normals, as well as many people with traumatic brain injury and other diseases, measuring the amount of energy in the different frequencies that we’re talking about. So we have a big database of patients, of normals and of patients. Again, this is superimposed on a century of knowledge of EEG. We didn’t invent the idea of slow waves. It’s just that we’re more sensitive; with MEG, I can see where the slow waves are happening. So then if you know where it’s happening, maybe there’s a way to treat it. Mingxiong will talk about the work we’re doing with actual treatments of these patients as well.
RAO: Perfect segue. And maybe you could also walk us through how long it takes. What’s the time course from when you gather the data to when you have the understanding?
HUANG: First of all, for the MEG research on mild TBI or concussion, we have been supported by the Department of VA for over 10 years to develop an MEG-based marker for making an objective diagnosis of mild TBI or concussion. Roland mentioned that, in the mild TBI case, the structural imaging—CT, MR—is not very sensitive. You can see maybe 5% positive findings. So we have been supported by the VA to develop MEG-based markers for that. And one of the markers Roland mentioned is the slow wave.
You can think about a slow wave like a local sleepiness. We’ve all heard about a slow wave or delta wave. They are the waves with about one to two hertz oscillation, rhythm, in the brain. We don’t see that when we are awake. But when we fall asleep every night, there’s a stage, called non-REM, deep-sleep stages three and four, when in the brain, the dominant signal is the delta wave or slow wave.
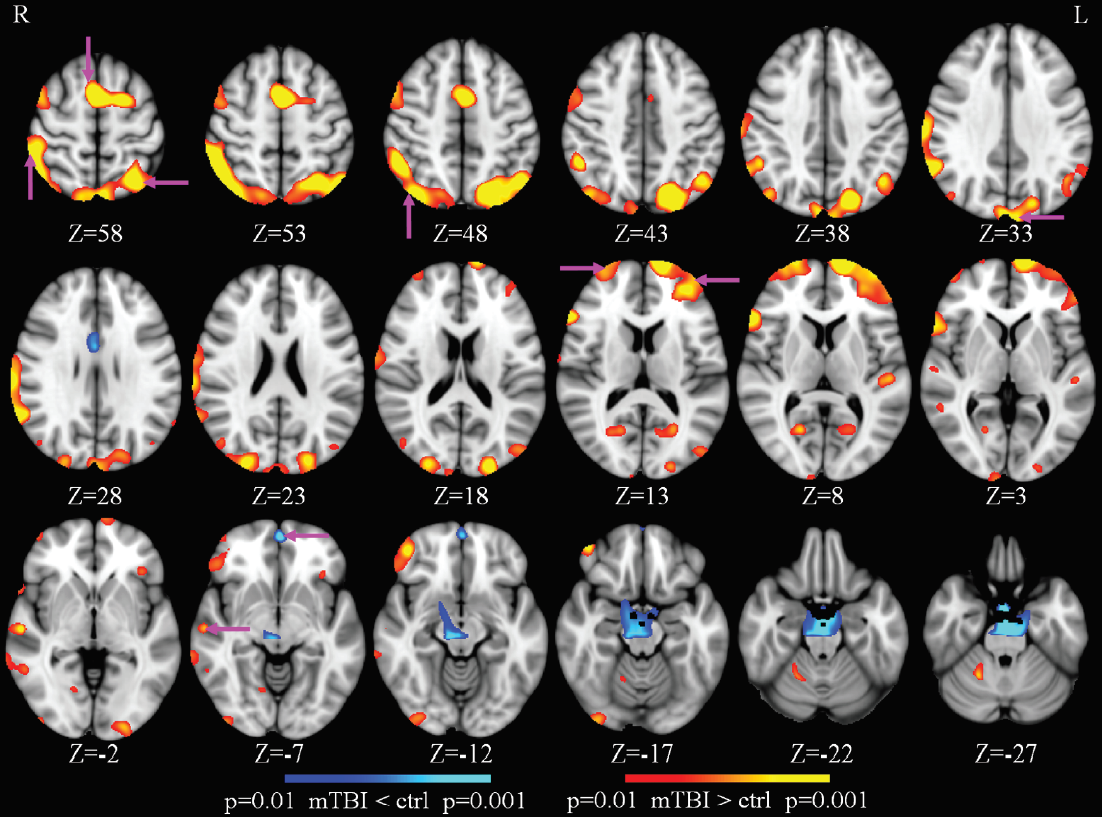
During that period of time, the brain is doing something fascinating. The brain actually opens up the cell space and cleans up those toxic chemicals like beta amyloid. So the delta wave is an indication of a metabolic clearance in the brain. In the case of TBI, even though patients are awake, there’s damage in the brain, and the brain tries to do the repair, for healing. So when we talk about the focal delta wave generation in the resting state when the subject is awake, we’re talking about local sleepiness, as the brain tries to repair the damage. That’s the signal work we’re picking up with MEG with good sensitivity for making a diagnosis of mild TBI.
Another marker we’re finding recently is a huge increase in the high frequency, the gamma band, which is about 30 to 80 hertz. That is a different mechanism. Roughly, there are two types of neurons. One is excitatory, the pyramidal cells, and they are sending the signals. Those are the ones that generate most MEG or EEG signals. But those signals are modulated by another type of neuron, smaller, called the GABAergic inhibitory neuron. Those GABAergic inhibitory neurons inhibit those big cells. When there’s concussion or mild TBI, it is likely the small type of cell is damaged. As a result of that, there’s a lack of inhibition. So imagine a little bit of noise in the background. The big cells start to fire. And when they fire, they generate high-frequency gamma band signals, 30 to 80 hertz.
So a combination of the low-frequency slow wave with this increase of normal gamma band activity allows us to make a good measurement of what part of brain actually is damaged by TBI.
LOOKING INTO THE FUTURE
RAO: Wonderful. That opens up so many new possibilities. I personally enjoyed watching the chamber being built every day as I walked in, but what are the prospects of being able to do this in free living conditions? Will you be able to make these sorts of measurements using new sensors without being in such a heavily shielded chamber so you can gather more data on what people are coping with as they recover from some of these conditions?
HUANG: As I mentioned, the reason we need this big magnetic-shielded room, the chamber, is because the MEG signals are weak. But there are some new developments. One of the developments that we have been following is a new type of sensor called an OPM, the optical pumping magnetometer.
Among the OPM sensors are two types. One of them is equivalent to what we call the amplitude modulation, like your AM radio. Another type of OPM sensor looks like FM radio, frequency modulation. Basically, they modulate data frequencies of the OPM sensors, taking measurements of the brain signals. Most types of OPM sensors are laser based.
There’s no liquid helium involved. So for the FM-based OPM sensor, there’s a recent publication; they can actually perform the measurements in the open field, without shielding. There’s still a long way to go before it’s available for clinical application, but it’s highly promising. Maybe in the future we can use a new generation of the OPM sensor with no shielding or medium shielding, and that can bypass one of the major limitations for MEG.
RAO: And these OPM sensors are small little devices, these optical pump magnetometers?
HUANG: Yes, the OPM sensor is the size of my thumb, a thumbnail. So it is very small, and you can put one anywhere you want. Almost like the EEG electrode, the OPM sensor can be put anywhere you want. So, number one, that reduces the gap between the source and the sensor. Currently in our MEG system, there’s a rigid helmet, so it’s one-size-fits-all. So there are always some gaps between the scalp and the helmet. But with the OPM sensor, you can have no gap between the sensor and the scalp. And, number two, you can develop the OPM sensors so the subject can move around. But there’s still a way to go before that becomes mainstream in MEG clinical and research applications.
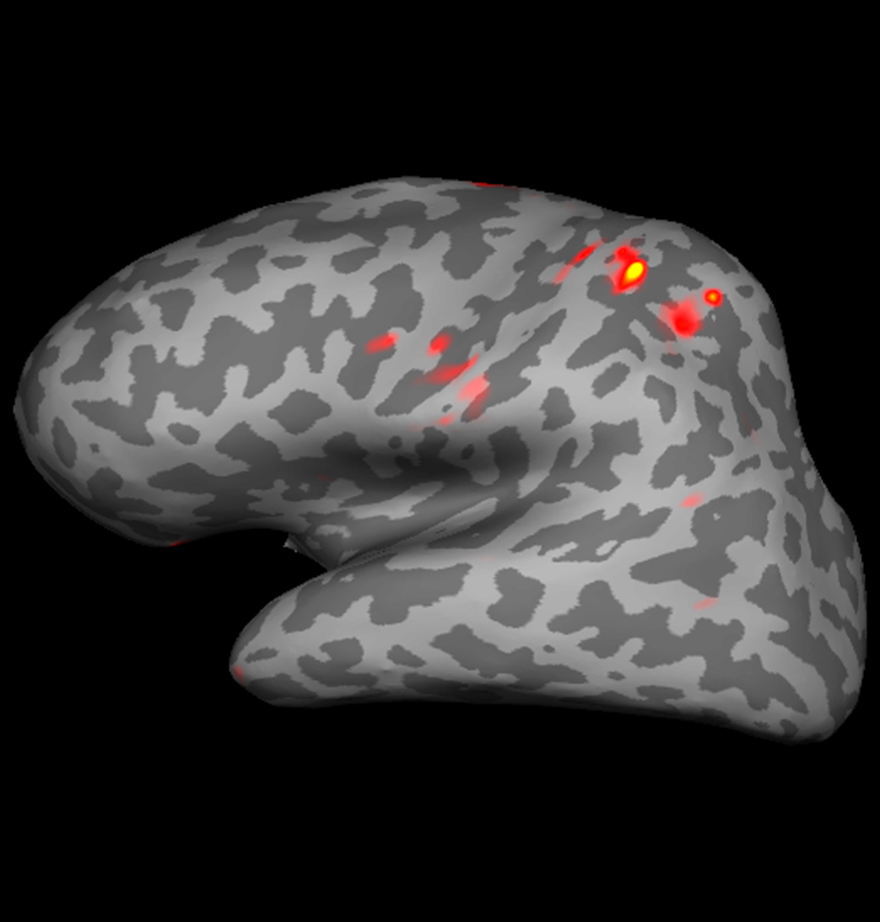
RAO: Yes, but it’ll happen sooner than we imagine. Twenty years ago there were no MEG machines, and here we are. So you got into this, Mingxiong, a bit, which is brain-computer interfaces. It’s fascinating. You said 20 milliseconds before you move your finger, there is a signal that registers. So do you see a future for using magnetoencephalography, especially the wearable context, as brain-computer interfaces for various applications?
HUANG: Yes. So we have a paper published recently, and the first author is Troy Bu (Yifeng). In that particular paper, we talk about using an MEG brain-computer interface to decode very subtle hand gestures, like rock, paper and scissors. Those were very challenging in the past. In the past, people used EEG for a brain-computer interface. Some people actually used invasive technology, like ECoG [electrocorticography] with electrodes, opening the skull and putting them on the cortex. You’ve probably seen some videos from Neuralink—Elon Musk and one of his companies—on monkeys.
But we are talking about noninvasive brain-computer interface. In the past, there’s EEG. For EEG, you can distinguish the motion from left hand and right hand, but decoding the subtle hand gestures of the same hand, like rock, paper, scissors, had been quite challenging for scalp EEG. But in that paper, with MEG we can approach 85% accuracy to decode three hand gestures, rock, paper, scissors.
Potentially, one day next-generation MEG sensors will be available, like OPM. If they can record the signal in a non-shielded environment, that opens the door. You can have a true brain-computer interface, noninvasively, based on magnetic signals, try to drive a robotic arm and also type on a keyboard. So we’re still in the early phase of trying to understand the potential of MEG-based noninvasive brain-computer interface, but in my opinion, that’s a very promising field.
RAO: So a baseball cap with these OPM sensors might be an effective way in which you can do BCI [brain-computer interface] work.
HUANG: Yes, that’s what we can imagine will happen in the future.
RAO: Wonderful. So, Roland, let’s pretend it’s 10 years out into the future. What do you think the state-of-the-art will be? Where will the developments that have been taking place lead us?
LEE: I think that one major development will be the optic pump magnetometers. The signal-noise that we have right now, though, cannot be excelled. The SQUIDs are just more sensitive than the OPMs, but it’s being shrunk. So the OPMS can get placed closer to the to the brain, the scalp and the brain, than the MEG, so that that does give them an advantage. But I don’t think the whole-head superconducting quantum interface will be replaced. That will be the gold standard. We can work things out, refine things. Liquid helium is expensive and it’s necessary for the superconducting quantum interference device; to get away from the liquid helium I think is really a major advance. Plus the fact that it can be therefore made more portable, and also it will be significantly cheaper.
RAO: So what will that help us do? Diagnose more diseases in the field? What crazy new things do you think will open up if that becomes a reality?
LEE: Well, possibly, for example, concussions, traumatic brain injury. Right now to do the MEG, they have to be flown to an MEG center. With that, you can have like a bicycle helmet. You put it on, and you can measure the slow waves. They’re doing similar things with the EEG. But of course, as we’ve mentioned, EEG is not as sensitive.
RAO: The smearing effect.
LEE: That’s right. Whereas with MEG, you can just have the sensitivity, without having to be flown to a special laboratory.
STATE-OF-THE-ART MACHINE, STATE-OF-THE-ART CHAMBER
RAO: So let’s turn a little bit to the nuts and bolts of the installation that we have here, that we are hoping to engage with a larger set of users. Mingxiong, so there is a chamber, and there is a machine. I was going to ask you to describe the chamber. You already mentioned it’s two feet of shielding, but what exactly is it? And, at the end, what did the chamber turn out to be in terms of its isolation? You said 70 dB isolation, but could you walk us through what the chamber is actually like from an engineering perspective?
HUANG: Yes, so the chamber we have is a six-layer magnetic shielding room made by a medical company from Switzerland. Among the six layers, three layers are called mu-metal. Nickel is a major part of that. Mu-metal actually blocks the electric-magnetic noise in the low frequency range, anything below 1 hertz. On top of that, there are three layers of aluminum with gold coating. Those actually block the noise above 1 hertz. So it’s a nice combination of mu-metal and aluminum with gold coating. The thickness is two feet, X, Y, Z. The MEG system is inside that. That provides excellent shielding not only for MEG, but potentially for other applications. Any experiment that requires an environment quiet in terms of electric-magnetic noise reduction, the chamber is actually ideal for that.
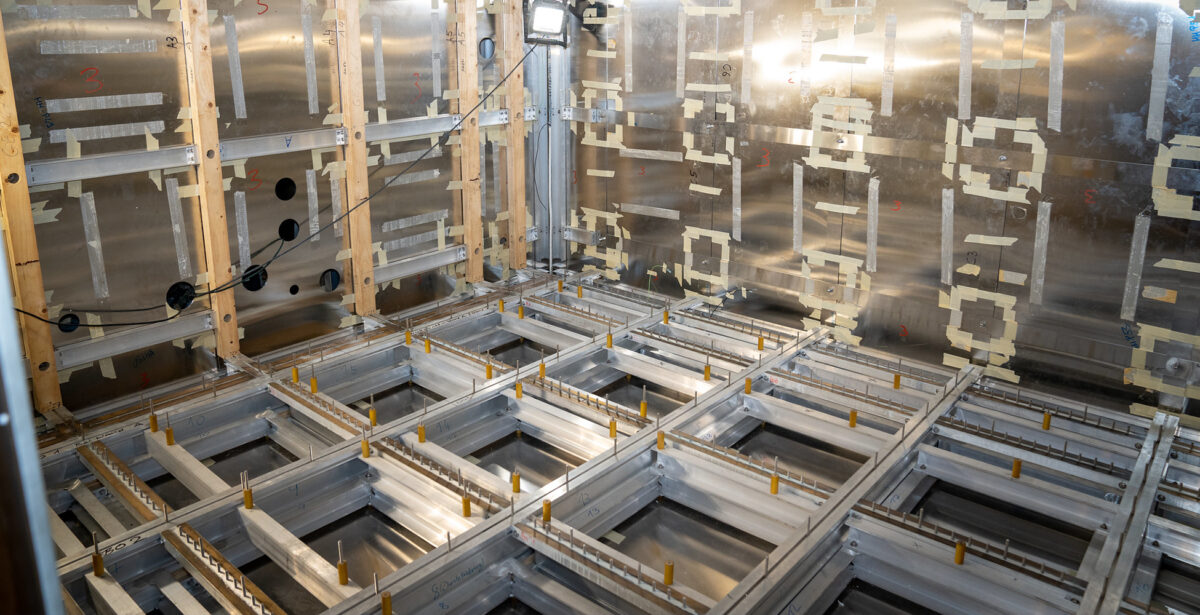
LEE: Just to note, most MEG units have, like, four layers of shielding. This has six, so it significantly improves the signal-noise. Of course, it also increases the thickness of the walls and the cost, but we’re luckily we have it.
RAO: So are these chambers common or do we have a small number of them around the nation? What’s your sense?
HUANG: So far, I think there are three of those of those chambers available for MEG.
LEE: With six layers.
HUANG: With six layers. One in Mass General Hospital in Boston and ours, and the third one I believe is in Israel. They’re making another one for a society in Shanghai, in China. So for the six-layer room, it’s very limited.
RAO: So there are only three locations globally?
HUANG: That’s right. So far. So that’s a unique facility on the floor, not only in recording the MEG signal but for basic science research.
RAO: And you had hard measurements made on how much the isolation was right, inside the chamber?
HUANG: Yes. So, based on the data we have, the shielding factor could be as high as 70 dB, which is very high, for low for the low frequency range. It goes higher for high frequency ones. That’s really the ideal environment for us.
RAO: Right. Recently, both of you won the NIH award that allowed you to procure a new MEG machine, just installed. So where does that position us regionally in terms of having this new advanced instrument?
LEE: We’re very fortunate. We came here in 2004. Within a year, in 2005, we had an up-and-running system in the screen room. It was in Sorrento Valley, which is like three miles north of the campus. But now we have the new machine right here in the School of Engineering, in Qualcomm Institute. The NIH grant enabled us to buy the newest version of the machine. It still has 306 sensors. But, of course, over the last 20 years, they’ve improved. The electronics are faster. The signal-noise is better. The noise suppression is better. So it’s an improved instrument. It’s the top-of the line instrument that exists in the top-of-the-line room. We’re very fortunate to be here with a really great instrument in a great environment.
RAO: So the patients that you’ll be able to run through this facility would come from all over Southern California? What’s the region that you think this facility will be able to serve?
LEE: Yes, right now there are only two MEGs in California. There’s one in UC San Francisco, and there’s one in UCSD, here. And so, historically, we have drawn patients from all of Southern California and neighboring Arizona. Of course, our own UCSD Medical Center sends some patients here. Rady Children’s is the only children’s hospital in San Diego; we get their patients. And we’re also drawing patients from the children’s hospitals in Los Angeles and Orange County, plus other major academic centers–UCLA, UC Irvine… and the other hospitals in town. Kaiser sends us a lot of patients. So we’re fortunate to be able to serve the patients throughout Southern California, and help them have the benefits of this unique instrument.
THE POWER OF PARTNERSHIPS
HUANG: For this NIH S10 grant, we’re very grateful. There are about 20 PIs who actually provided support for us to get this S10 grant. Without their support, there’s no way we could receive this award. Those PIs actually work in different fields, from basic science research to different clinical applications. We’d be glad to have them use our machine for different research projects.
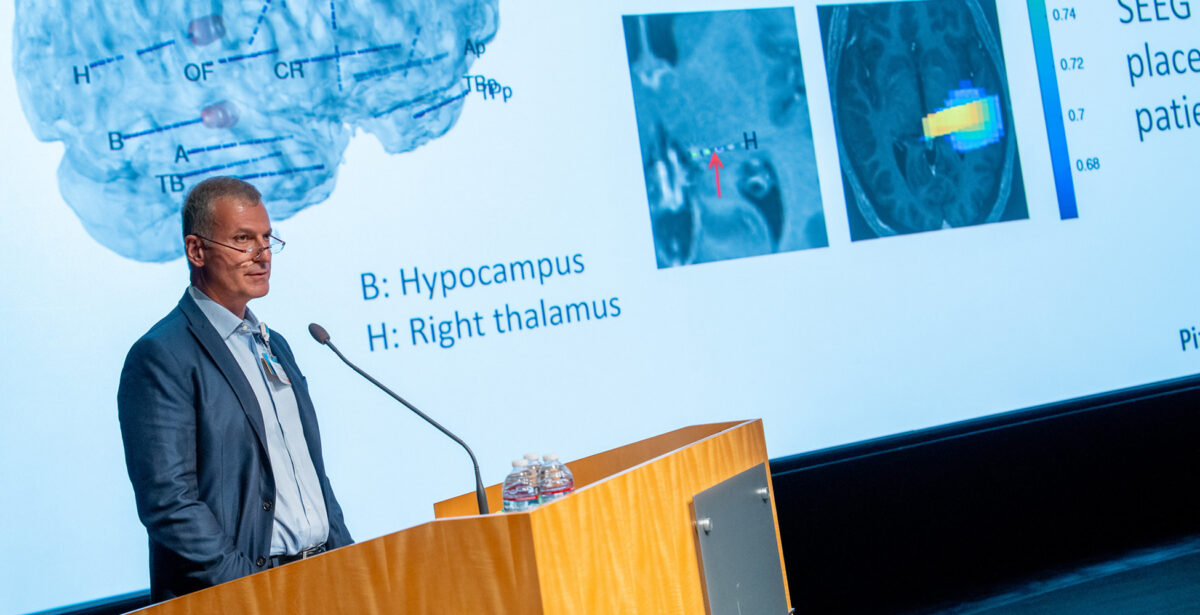
RAO: So you welcome new partnerships?
HUANG: Absolutely. This new MEG machine is called the TRIUX neo, and the sensor configuration Roland mentioned is pretty much the same sensory configuration as our previous machine. So that allows us to merge data sets together. So if somebody collected the data from the previous machine, the sensor configuration is pretty much identical. So that allows us to group them together in a bigger database.
The new machine has a closed helium recycling system. So we don’t need to refill the helium every week, only once a year during annual maintenance. This solves the problem of the operational cost because, helium prices go higher and higher. And the signals from the new machines are excellent.
LEE: One thing I would like to emphasize is the NIH funded this machine as a research machine. There will be a lot of research that will be done on the machine to satisfy the NIH’s requirements. And we are lucky at UCSD to have a large range of collaborators that have been working with us, and we’ll get more of those.
The first author, Troy Bu, that we mentioned—that’s a grad student here in the ECE [Electrical and Computer Engineering] and CS [Cognitive Science] Department[s] at UCSD. So we’re fortunate to have such a talented pool of students and postdocs to work with us.
RAO: It’s been wonderful to see the collaborations develop. So now inside the chamber you’re of course monitoring the signals from the head. You have ways in which you can stimulate the median nerve, but you can also visually stimulate the individual. You have a screen in there?
HUANG: Yes. So for our center, we offer different ways to activate brain function. The median nerve, tactile sensory stimuli, that’s something we provide. Also we have a response pad for monitoring the motor response. We have auditory, a left and right auditory system. We mentioned we have a high-resolution video monitor projector screen in front of the MEG. So that allows us to present words, pictures, movies; that offers multimedia, a way to activate brain functions. We’ll be working with any PI who has an interest in developing new protocols, for example, for working memory. In a different population, we’re looking at traumatic brain injury. We also have been funded by the VA, looking at other disorders like post-traumatic stress disorder, PTSD. Mild TBI and PTSD are major health issues for our veteran population.
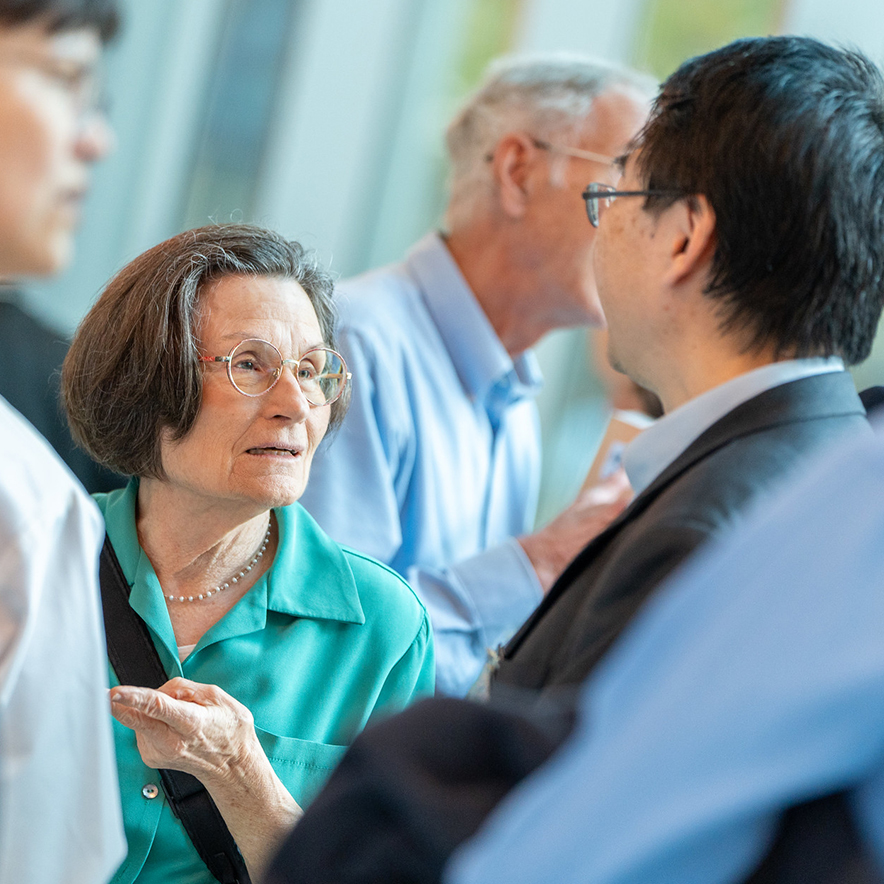
In the case of PTSD, we’re looking at the PTSD neural network. The major components there are the amygdala, which is the part of the fear network that works together with the hippocampus, part of the memory network. And there’s another area called the ventral medial prefrontal cortex, VMPFC. The VMPFC behaves like a brake when driving a car. The amydgala is like the engine. When our veterans are exposed to trauma and have a fearful memory in their system, the VMPFC can send down top-down modulation to suppress the fear. But when there’s damage, maybe due to co-morbid TBI because our veterans are exposed to blasts a lot, the VMPFC can be damaged. That means less top-down modulation. That may explain why, in veterans with existing mild TBI, their PTSD is the greatest, double or triple.
RAO: The propensity for suffering from PTSD later on?
HUANG: Exactly. That is actually documented by some excellent research from a long-term collaborator, Dr. Dewleen Baker. And so the MEG provides us an excellent functional imaging tool to study mild TBI, PTSD. We’re also interested in the early Alzheimer’s disease, other disorders, also some normal or abnormal brain functions, in general.
RAO: It’s wonderful. It’s been a great conversation. I should say that, from the Qualcomm Institute’s point of view, we were set up as an Institute for Science and Innovation, and part of that meant creating new infrastructure that would be the watering hole around which new researchers could come together. And so we’ve always been on the lookout for new ways to enhance our capabilities. And this is the most recent one. It’s been delightful, as you point out, to have a student from Electrical Engineering and Computer Science and Cog Sci connect with it at every level, from experimenting with the OPMs to doing machine learning algorithms, to expediting the process of scoring these spike signals that you get. We are hoping that this will be the first opening move in a whole new set of conversations and new set of collaborations that emerge. We’re hoping that this facility brings together many more people than you already have. It’s been great to have the two of you share some time and your thoughts. Thank you.
LEE: We’re very excited and grateful to the Qualcomm Institute. Again, we’re looking forward to many collaborations, and it’s a very exciting time. Thank you very much.
HUANG: We’re so grateful, Ramesh, you allowed us to come over here, to set up the MEG Center over here. I think that’ll be a great journey from now on. Thank you so much for allowing us to move, relocate our lab over here. Thank you so much.
RAO: Thank you. And a pleasure.
For more information about the MEG Center, visit its QI web page or the MEG Center website. In addition to directing the MEG Center, Lee is emeritus professor of radiology, UC San Diego School of Medicine and physician with Veterans Affairs (VA) San Diego Healthcare System; Huang is adjunct professor with the UC San Diego ECE and Radiology Departments and scientist with VA San Diego Healthcare System.